Dr. Thomas Davidson - Rutgers University
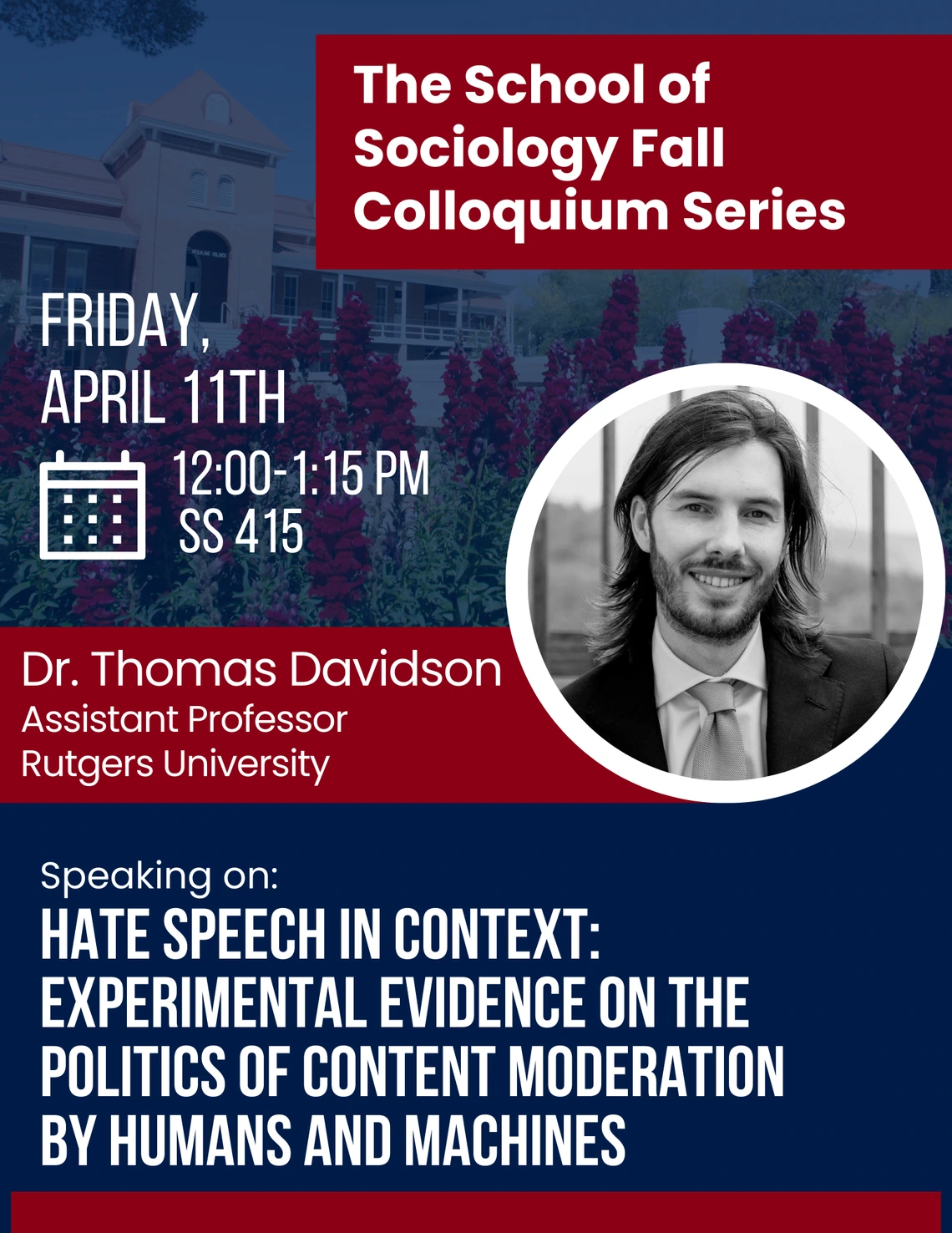
When
Where
Hate Speech in Context: Experimental Evidence on the Politics of Content Moderation by Humans and Machines
Social media platforms routinely employ workers and automated systems to moderate online content at scale. While these systems can help remove offensive, misleading, and inflammatory material, they can also suppress legitimate speech and discriminate against minority groups. This work aims to advance the sociology of content moderation to understand why large-scale systems can generate to unintended discrimination. I examine this problem by using a conjoint experiment to analyze how people evaluate online hate speech. I assess how perceptions of hate speech vary according to characteristics of the target, the speaker, and the observer, as well as other contextual factors. The results show a general consensus about the speech most likely to offend or violate policies. Posts with racial slurs from White users are typically rated as the worst, followed by homophobic language. However, there is heterogeneity among observers with respect to race, ideology, and attitudes. Notably, whites, conservatives, and those scoring high on racial resentment scales tend to flag so-called ``reverse racist'' language by Black users at higher rates, highlighting disparate understandings of hate speech. Survey evidence provides further insights into how experiences of hate speech and attitudes towards it vary markedly across demographic and political axes. In a second study, I repeat the experiment using state-of-the-art vision-language models to test whether artificial intelligence systems use context in similar ways. I find that the largest models mirror some aspects of human decision-making, suggesting that these tools could help to improve automated content moderation, but draw attention to persistent biases and disparities.